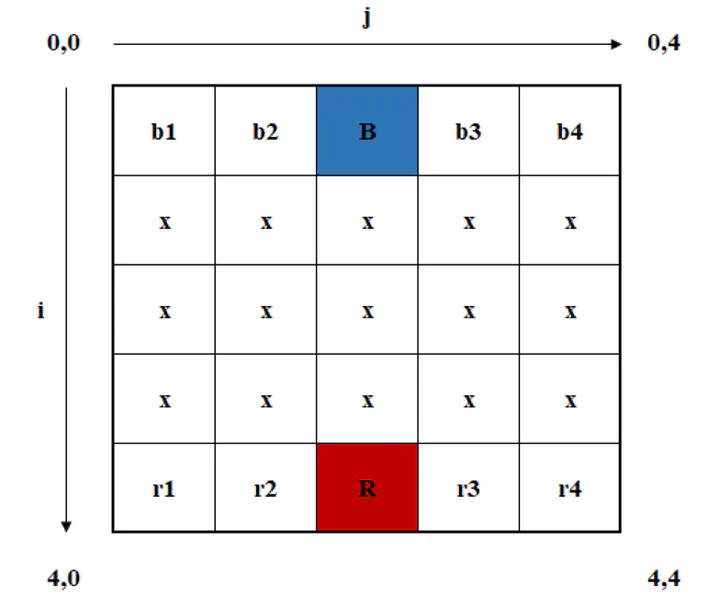
This project showcases multiple traditional and Deep RL approaches to creating an artificial agent that plays Onitama proficiently. The Minimax Depth 5 and above models were found to be unbeaten by any human that competed against it.
The following algorithms were Implemented:
- Minimax with Alpha-Beta Pruning (single and multi-processor versions)
- Monte-Carlo Tree Search
- Deep Deterministic Policy Gradient
- Deep Double Dueling Q Networks
The full details and investigation of the implementations of the algorithms 1 & 2 can be found in TraditionalAI_Report.pdf
and the details for algorithms 3 & 4 can be found in DeepRL_Report.pdf
Setup
To set up, clone this repository and have a Python 3.6.9 environment with the following libraries installed:
torch==1.8.1
numpy==1.18.5
seaborn==0.11.1
matplotlib==3.3.3
tqdm==4.59.0
torchsummary==1.5.1
This board game is set up as a Markov Decision Process (MDP).
Traditional AI Gameplay
To play against the Minimax and MCTS agents, run the play_onitama
function form play_onitama.py
play_onitama(game_mode, first_move = None, verbose = 1, minimax_depth =
None, minimax_depth_red = None, minimax_depth_blue = None, aivai_turns =
500, timeLimit=None, iterationLimit=None, iteration_red = None,
iteration_blue = None, mcts_efficiency = "space", parallel = None)
The game_mode
controls the type of the two players. It can be a minimax vs minimax or a player vs MCTS and so on. The strengths of the AIs can be set in the other parameters.
Deep RL Training
To train the Deep RL agents, go to Train.py
and adjust the parameters in the onitama_deeprl_train
function. An instance of the function is as shown below:
onitama_deeprl_train("train", "DDPG", 10000, "insert_training_name", "minimax", 1,
discount_rate = 0.99, lr_actor = 0.001, lr_critic = 0.001, tau = 0.005,
board_input_shape = [10, 5, 5], card_input_shape = 10, num_actions = 40,
max_mem_size = 1000000, batch_size = 128, epsilon = 1, epsilon_min = 0.01,
update_target = None, val_constant = 10, invalid_penalty = 500, hand_of_god =
True, use_competing_AI_replay = False, win_loss_mem_size = 1000,
desired_win_ratio = 0.6, use_hardcoded_cards = True, reward_mode =
"simple_reward", minimax_boost = 1, mcts_boost = 5000, plot_every = 1000,
win_loss_queue_size = 100, architecture = "actions_only", moving_average = 50,
verbose = False, valid_rate_freeze = 0.95)
The algorithm can be set as either DDPG
or D3QN
. The competing agent used to train against the agent can be either minimax
or MCTS
. The Minimax or MCTS competing agent will boost its strength once in the last win_loss_queue_size
episodes, the DeepRL agent wins at a rate higher than desired_win_ratio
. The architecture
of the neural network (refer to DeepRL_Report.pdf
for the details) can be set as well.
One example of a neural network architecture used is the following, where the validity and actions branch separately and multiply to the final output layer. More details can be found in DeepRL_Report.pdf
.